Satellite images are becoming increasingly available. They now allow to monitor the characteristics of terrestrial vegetation with a good spatial and temporal resolution, which opens up many application perspectives related to biomass estimation, land use classification, water stress monitoring, and even prediction of commodity prices and food insecurity assessment.
However, the use of satellite images poses many methodological challenges concerning data analysis and the development of predictive models. In particular, the existence of spatial and temporal components, the very large size of the satellite datasets (increasing with the improvement of their resolution), or the correlations between vegetation indices derived from different types of satellite observations raise many difficulties. In this context, various technical solutions have recently been proposed to reduce the dimensions of datasets derived from satellites, while preserving a maximum of information.
The objective of this workshop is to present recent research works with a substantial data analysis component (statistical analysis, machine learning, data visualization etc.) and related to the analysis of satellite products to monitor the states of terrestrial vegetation. Each presentation will focus on a specific vegetation monitoring objective and present a range of technical solutions to analyze large datasets derived from satellite observations.
ABSTRACTS
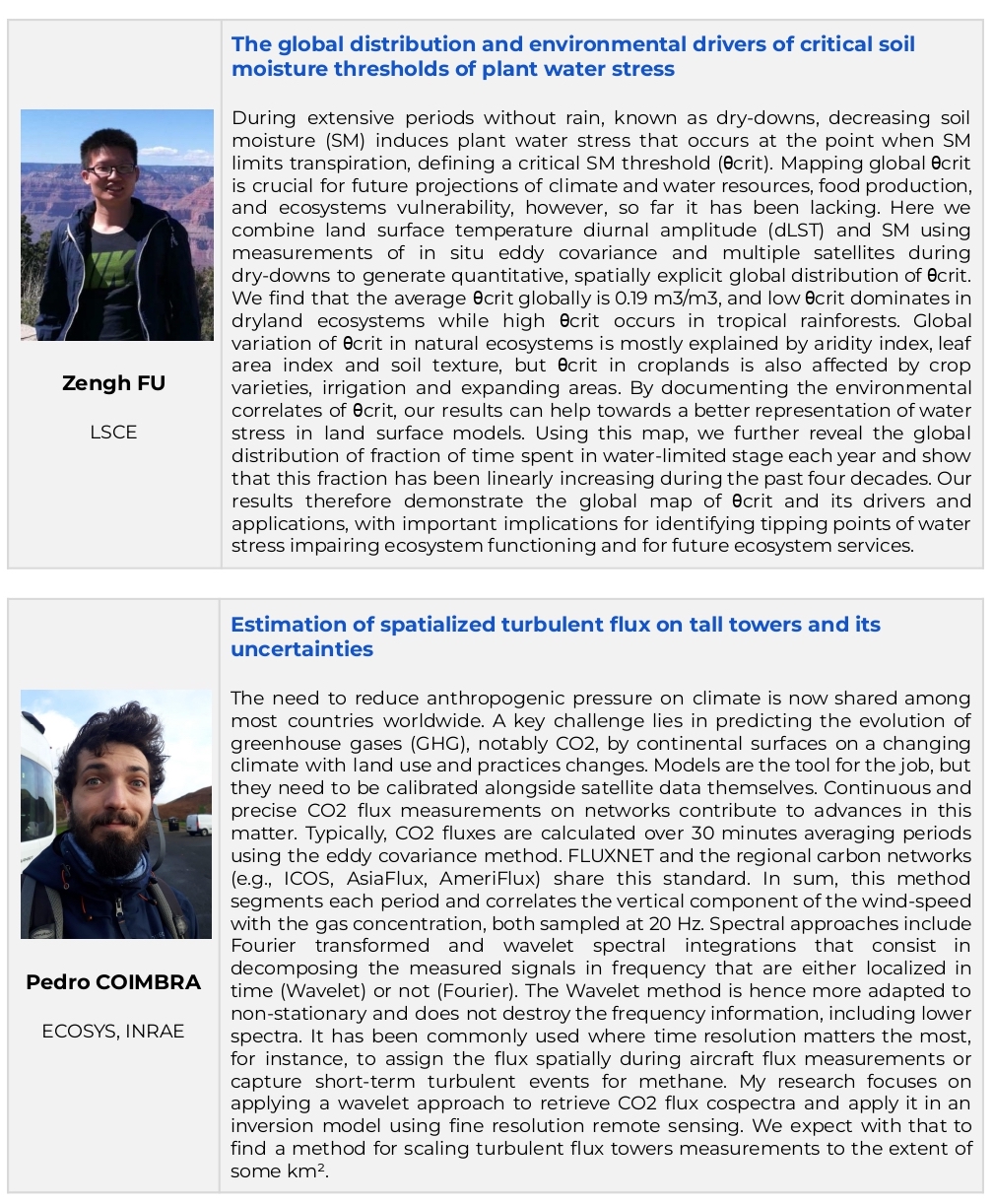
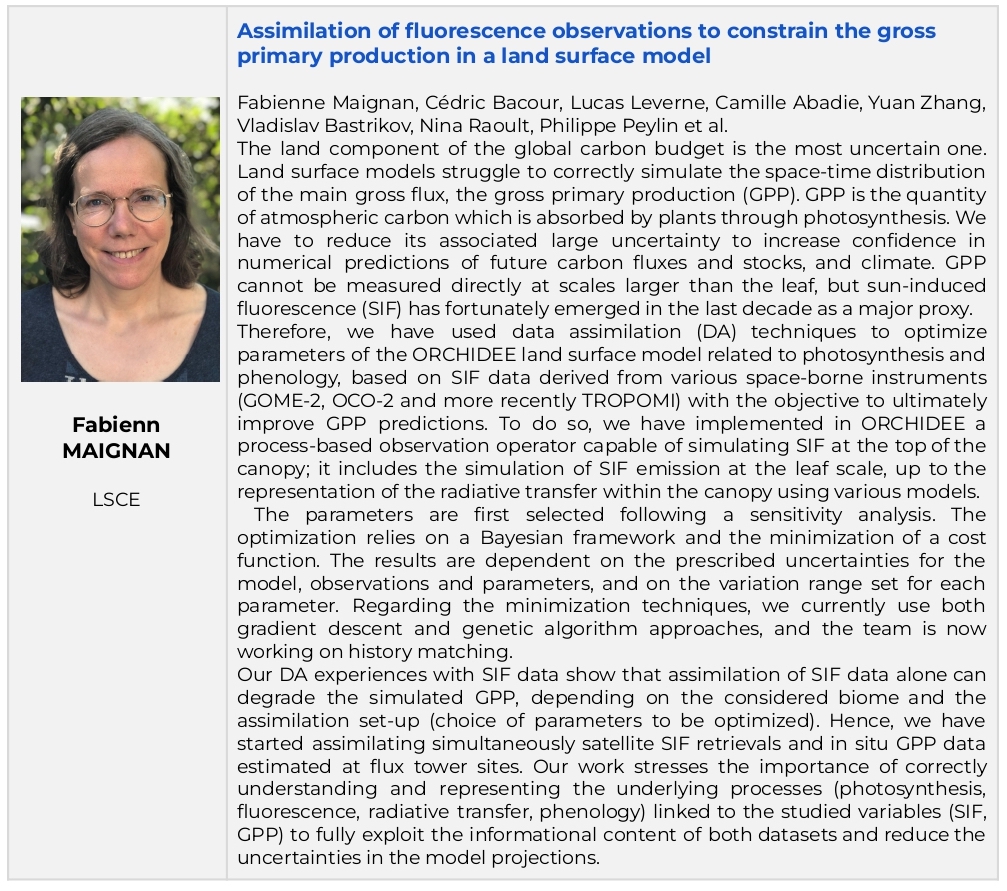
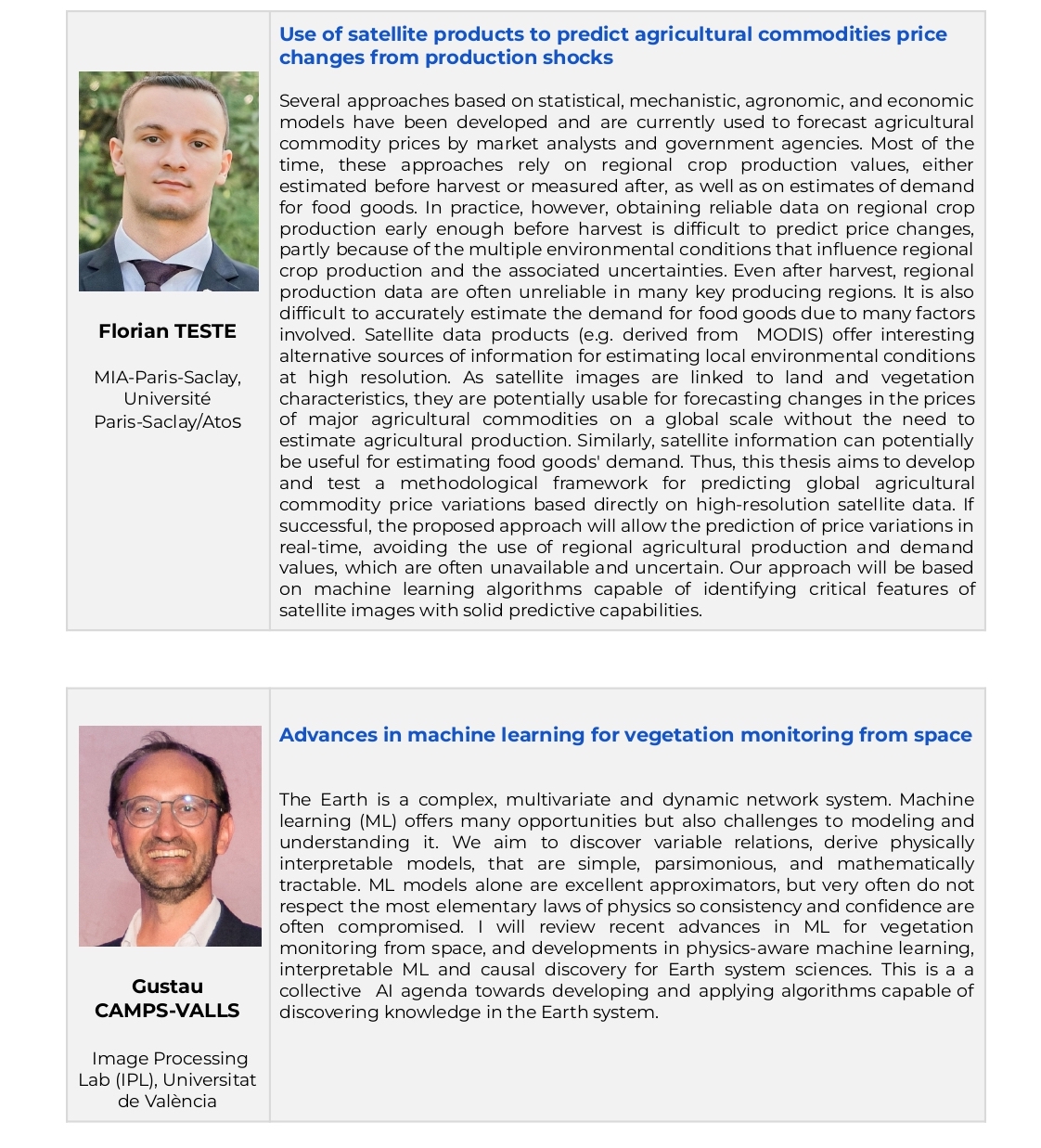
Global critical moisture threshold of plant water stress
Zheng FU
(Researcher at LSCE)
Estimation of spatialized turbulent flux on tall towers and its uncertainty
Pedro COIMBRA
(PhD at INRAE)
PDF
Assimilation of fluorescence observations to constrain
the GPP in a land surface model
Fabienne MAIGNAN
(Senior Researcher at LSCE)
PDF
Use of satellite products to predict agricultural commodities
price changes from production shocks
Florian TESTE
(PhD at MIA, UP-Saclay)
PDF
Advances in machine learning for vegetation monitoring from space
Gustau CAMPS VALLS
(Senior Researcher at Universitat de Valencia)