Machine learning for the study of climate and its impact - December 8, 2020
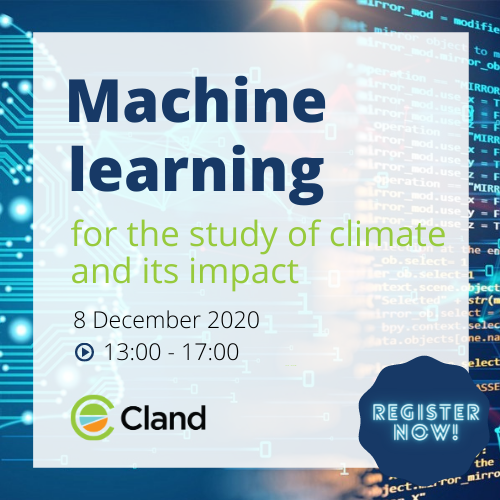
CLand Convergence Institute has organized on December 8, 2020 by videoconference a workshop dedicated to “Machine learning for the study of climate and its impact”.
Machine learning is increasingly being used in climate science for a variety of applications, including greenhouse gas emissions, agricultural yield forecasting, mapping plant and soil characteristics, and emulating process-based models. This workshop brought together ten international speakers who presented machine learning applications to solve important problems related to climate change.
Find all the workshop presentations below
Please note that the videos and presentations available here are protected by a CC-BY-SA license.
This means you may not use the material for commercial purposes and must give appropriate credit.
More information
Machine learning as a framework for spatial and spatiotemporal
prediction in environmental and earth sciences: the dos and don'ts
Tomislav HENGL
[PDF]
Machine learning to predict peatland greenhouse gas emissions
Yuanyuan HUANG
[PDF]
Creating spatial insights into global-scale model inaccuracies
Johan VAN DEN HOOGEN
[PDF]
The decreasing vulnerability of French crop production to climatic hazards
Bernhard SCHAUBERGER
[PDF]
Walking through a random Forest: how to build, select, and inspect
a model of global forest symbioses?
Brian STEIDINGER
[PDF]
The implementation of machine learning algorithms in the prediction of
crop production in conservation agriculture under climate change
Yang SU
[PDF]
Tracking methane emissions globally using hyperspectral imaging
Alex D'ASPREMONT
[PDF]
A machine learning method to understand the primary climatic
drivers of wheat yield shocks across Europe
Peng ZHU
[PDF]
Machine learning emulators reveal divergence in soil carbon
controls between observations and process-based models
Katerina GEORGIOU
[PDF]